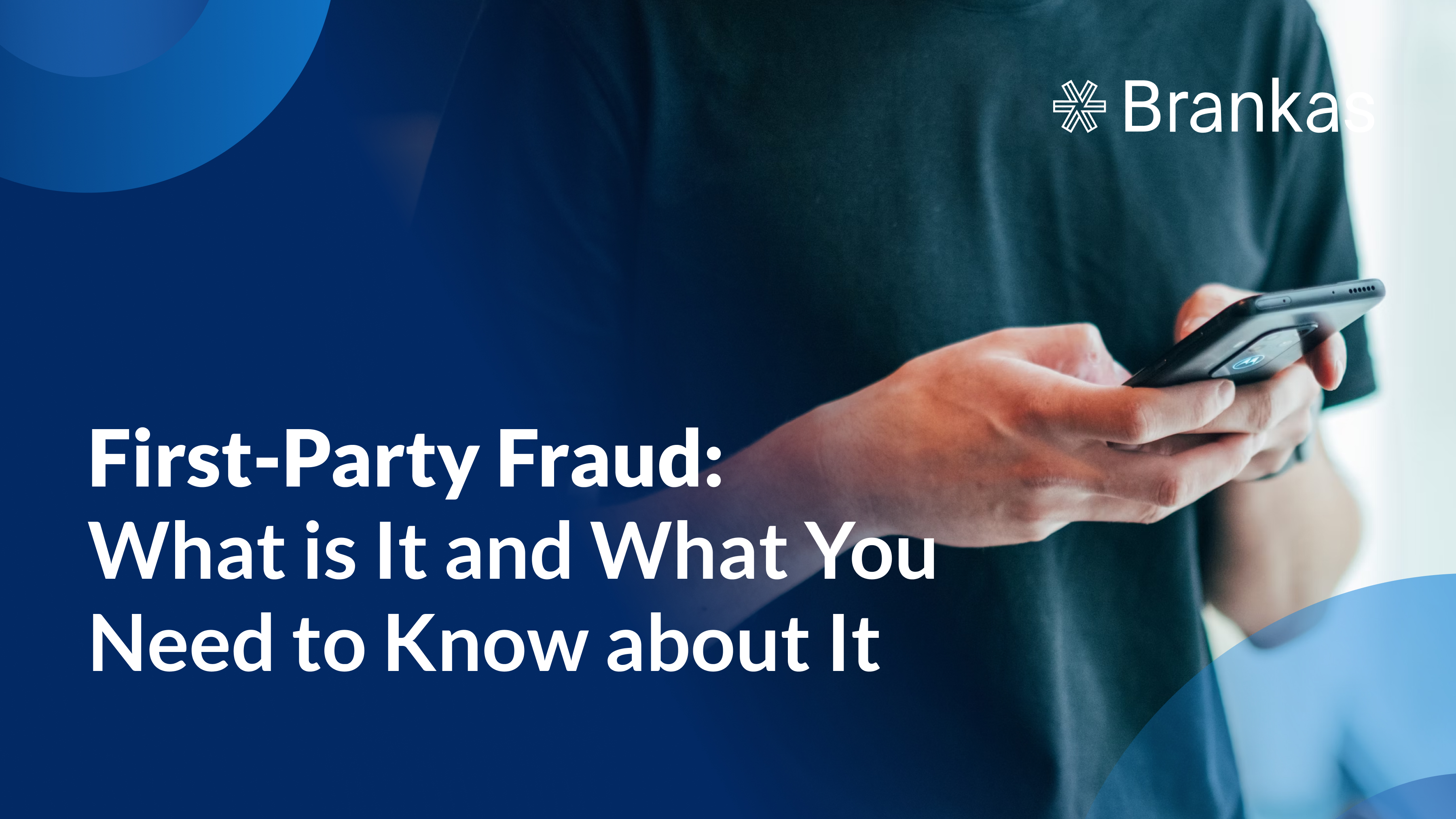
Understanding the intricacies of chargebacks and refunds is paramount for merchants. Chargebacks and refunds play crucial roles in customer satisfaction and maintaining a positive business image.
Credit scoring is a statistical analysis used by lenders and financial institutions to assess a person’s or a small business’s creditworthiness. Traditional credit scoring methods rely on limited data sources, such as credit reports and payment history. These methods often exclude individuals without established credit histories, like the unbanked and underbanked. As a result, many people face challenges accessing credit. Alternative credit scoring data sources are now increasingly used to determine a borrower’s trustworthiness.
Traditional credit scoring methods primarily depend on a narrow set of data and focus on a borrower’s past credit activities. These metrics provide some insights but they overlook other factors that could influence a person’s creditworthiness.
The unbanked, underbanked, and people who have avoided using credit products are often excluded from traditional credit scoring. They face difficulty securing loans or credit cards despite possibly having sound financial habits. This exclusion perpetuates a cycle where those needing credit to build their credit history are denied access, hindering their financial progress.
Traditional credit scoring models can also embed biases that disproportionately affect certain groups. Credit scores may be skewed against minorities or low-income individuals. Models that heavily weigh credit usage might disadvantage those who prefer to operate within a cash economy.
Credit scoring models enable lenders to assess the risk of lending money or extending credit. These are some alternative credit scoring models:
Machine learning and AI-based
Machine learning and AI-based credit scoring models analyze vast amounts of data to identify patterns and predict creditworthiness more accurately. Companies like Tala, Nubank, and Upstart use machine learning algorithms to assess credit risk. These models reduce reliance on limited data sources, enhancing the fairness and precision of credit scores.
Behavioral scoring
Behavioral scoring models consider spending patterns, social media activity, and other behavioral indicators. For example, regularly paying utility bills on time or having a stable job can positively influence a behavioral credit score. This approach provides a more comprehensive view of an individual’s financial habits and reliability. CreditXpert uses rent and utility payments. Kreditech analyzes social media profiles, online behavior, and smartphone data as part of their credit assessment.
Hybrid models
Hybrid credit scoring models combine traditional and alternative data sources to create a more robust assessment of creditworthiness. This combination helps address the shortcomings of relying solely on credit histories and enhances the accuracy of credit assessments. Kabbage uses a hybrid model that incorporates traditional credit scores and real-time business performance metrics from sources like accounting software, payment processors, and bank accounts. Zest AI combines traditional credit data with alternative data, including utility and rent payments, to create more inclusive credit scoring models.
A 2022 McKinsey survey of 44 financial institutions worldwide found that 60% have increased usage of alternate types of data. Sources may include:
1. Non-traditional financial data
A. Utility and rent payments- Regular, timely payments of these bills demonstrate financial responsibility and stability. Some credit scoring companies, like Experian Boost, already include such data to improve credit scores for individuals with limited credit histories.
B. Bank transaction data- Analyzing a borrower’s income, spending patterns, and saving behavior allows credit scoring models to gain a deeper understanding of an individual’s financial health. This approach can help identify creditworthy individuals who might not have a robust credit history but manage their finances well.
C. Insurance and telecom payments- Regular payments for insurance policies or mobile phone bills indicate financial discipline and commitment. Companies like Nova Credit are pioneering the use of such alternative data sources to offer more inclusive credit scoring solutions.
2. Digital footprint data
A. Social media and online activity- Analyzing patterns such as social connections, professional networks, and online interactions can help assess creditworthiness. Some fintech firms, like Kreditech, leverage social media data to enhance their credit scoring models.
B. E-commerce and mobile payments- Frequent, consistent use of digital payment platforms can indicate financial stability and trustworthiness. This approach can expand credit access to tech-savvy individuals and those preferring digital transactions.
Using alternative data sources for credit scoring raises ethical considerations.
One serious concern is privacy and data security. There is a heightened risk of sensitive information being mishandled or breached with the integration of alternative data sources. Financial institutions must implement robust data protection measures to ensure consumer information privacy. Compliance with data protection regulations, like the General Data Protection Regulation (GDPR) and the California Consumer Privacy Act (CCPA), is essential to protect consumer rights and build trust.
Fairness and bias in credit scoring models are also critical issues. Traditional models have faced criticism for perpetuating biases that disadvantage certain groups, such as minorities or low-income individuals. New models, especially those using AI and machine learning, must be carefully designed to avoid replicating or exacerbating these biases. Ensuring transparency in how credit decisions are made is crucial. Consumers should understand why they receive certain credit scores and can dispute inaccuracies.
The limitations of traditional credit scoring significantly impact credit access and financial inclusion. New credit scoring models and data sources offer improved accuracy and predictive power, enabling lenders to make more informed decisions. These innovations enhance financial inclusion by expanding credit access to underserved populations, including individuals without traditional credit histories. Advanced models, particularly those utilizing AI and machine learning, can be designed to minimize biases present in traditional methods, promoting fairness in credit assessments. The use of real-time data and advanced analytics facilitates faster and more efficient processing of credit applications, benefiting both lenders and borrowers. These advancements encourage responsible financial behavior and contribute to a more inclusive and efficient credit scoring system.
Financial institutions can create a more inclusive, accurate, and efficient credit scoring system that better serves a diverse range of borrowers by leveraging the benefits. Expanding credit access through innovative scoring methods is crucial for fostering inclusive growth, financial equality, and economic development.
Discover the power of Brankas lending APIs for enhancing your loan application process by tapping into alternative credit data.
Understanding the intricacies of chargebacks and refunds is paramount for merchants. Chargebacks and refunds play crucial roles in customer satisfaction and maintaining a positive business image.
Brankas is the first company to secure the PJP Category 2 License for Account Information (AInS) from Bank Indonesia, marking an open banking data milestone for Indonesia and giving businesses added visibility on payment transactions.